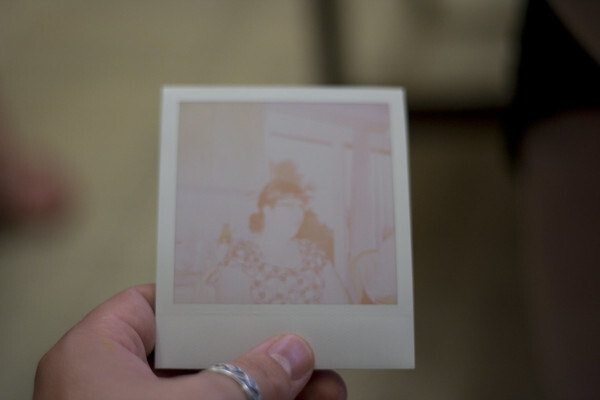
(JoshNV/Flickr)
A few weeks ago, CNN host Jake Tapper offered an excellent example of the problems with Twitter’s image-cropping algorithms.
Not that he was trying to do that, but sometimes algorithms have a mind of their own.
In an effort to show off who he was working with at the time, he took selfies on either side of the CNN anchor desk (where Dana Bash and Abby Phillip, respectively, were sitting)—and when Twitter got a hold of those selfies, the artificial intelligence decided to focus on what it thought was the focal point of the two images … which turned into an unintentionally hilarious moment on the Twitters for Tapper:
It wasn’t the only example of Twitter’s machine-learning algorithms getting in the way, but it was perhaps the most prominent recent one. And it was the perfect example to highlight a common criticism of the algorithm. Given the choice between highlighting a man and a woman—in one case, a woman of color—over two separate tries, the Twitter algorithm chose Tapper in both cases.
With that context in mind, it makes a whole lot of sense that, this week, the social network released information on research it had done to figure out whether machine learning, based on human eye-tracking data, was the best solution to this problem. In a blog post by respected ethical data scientist Rumman Chowdhury, the company’s director of software engineering, the company explained that it started using this algorithm in 2018 to offer more consistent photo sizes on the social network.
“The algorithm, trained on human eye-tracking data, predicts a saliency score on all regions in the image and chooses the point with the highest score as the center of the crop,” she wrote.
The research found that women were generally favored in photo comparisons between men and women, and that in comparisons between black and white individuals, the algorithm tended to favor white individuals.
The research also tested for “male gaze,” or the objectification of women by the algorithm, and found no evidence of objectification bias. However, Chowdhury said that the research raised broader concerns about an algorithm making the choice of cropping a photo at all. After all, there’s a reason this discussion comes up.
“Even if the saliency algorithm were adjusted to reflect perfect equality across race and gender subgroups, we’re concerned by the representational harm of the automated algorithm when people aren't allowed to represent themselves as they wish on the platform,” she writes.
And that led Twitter to stop cropping its photos in this way—something it recently started doing on its iOS and Android apps. Ultimately, machine learning gets things wrong sometimes, and it creates deeper issues of bias than unintentionally making Jake Tapper look like a prima donna. And the company embraced that lesson.
This is a great decision by Twitter and one that I hope finds interest in other areas of research—as decisions like these will ultimately help us find an ethical balance with all this machine-learning data in the years to come.
Thanks, Jake Tapper, for providing the perfect example of this problem in action.
Time limit given ⏲: 30 minutes
Time left on clock ⏲: 2 minutes, 11 seconds